Fine-Tuning: Bridging the Gap in AI Model Specialization
This week, we're zeroing in on fine-tuning – the powerful process that transforms a pre-trained AI model into a domain expert. Discover how fine-tuning bridges the gap between general knowledge and specialized performance, making AI truly adaptable and effective for specific tasks!
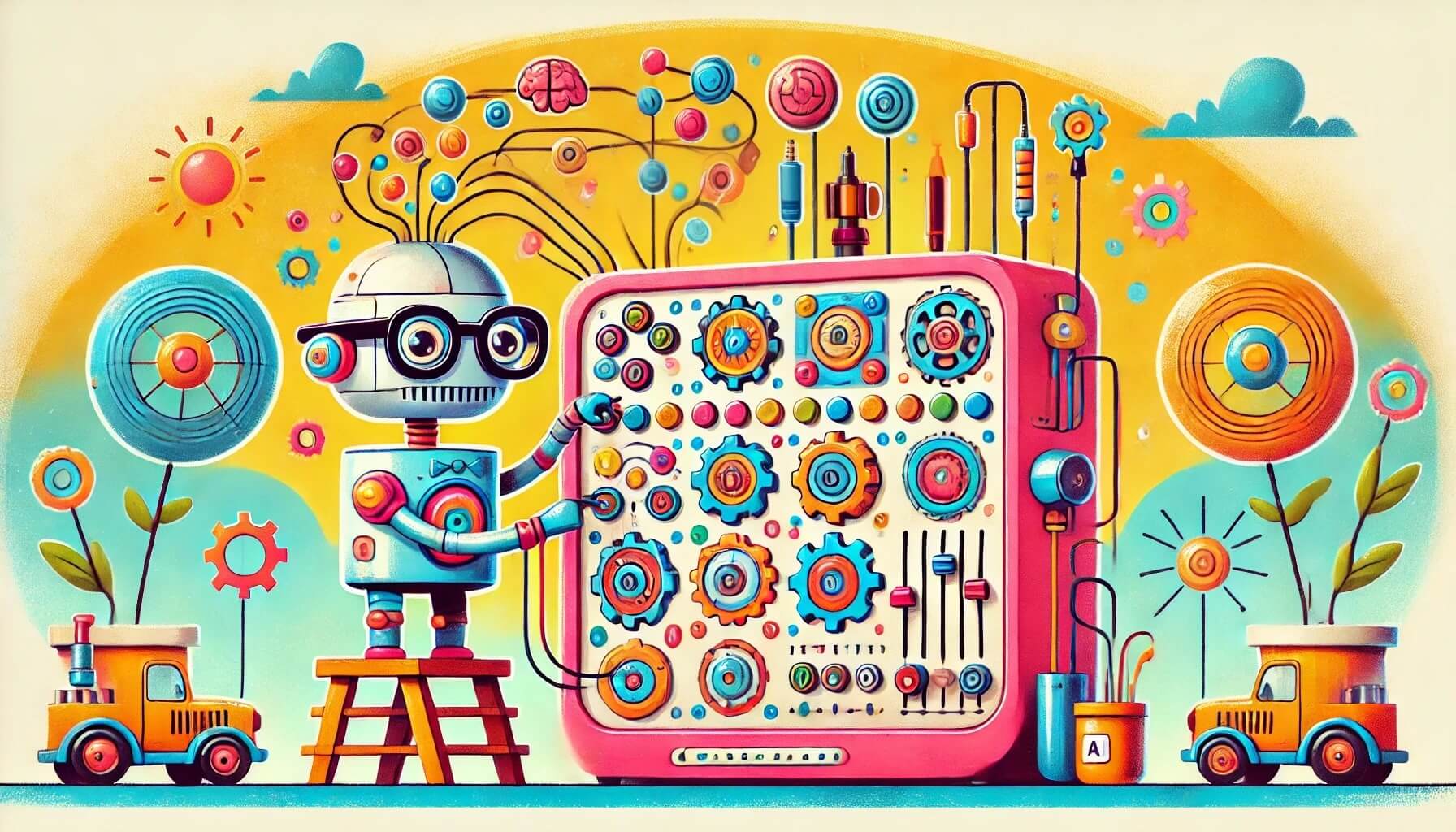
Understanding Pre-Training: The Foundation of AI Models
Pre-training is the crucial first phase in developing AI models, where models learn from vast and diverse datasets such as books, articles, and online content. During this stage, models capture fundamental language patterns, grammar rules, and contextual relationships. This broad-based training equips the AI with a general understanding of language, making it capable of handling various tasks. However, this general knowledge often falls short when applied to highly specific or specialized tasks, such as understanding medical terminology or providing expert customer support.
Pre-Training Alone Isn’t Enough
While pre-training gives models a strong general foundation, it lacks the nuance needed for specialized use cases. Imagine a general-purpose AI model being asked to assist with legal document review or to provide technical support in the software industry—it would likely struggle without a deeper, domain-specific knowledge base. Pre-training alone cannot fill this gap, as it focuses on capturing general linguistic structures rather than deeply understanding any one subject. This is where fine-tuning comes in as a game-changing next step in AI development.
Fine-Tuning: Tailoring AI for Specific Needs
Fine-tuning involves taking a pre-trained model and refining it on a smaller, targeted dataset specific to a given task or domain. By exposing the model to task-specific data, it adjusts its internal weights to improve accuracy and relevance within that context. Unlike the resource-heavy pre-training phase, fine-tuning is cost-effective and efficient. For example, a healthcare provider can fine-tune a language model on patient records and medical journals, enabling it to deliver more accurate diagnoses and recommendations tailored to a medical setting.
Real-World Examples: Fine-Tuning in Action
Fine-tuning has proven invaluable across various industries. Consider a customer service chatbot tailored for financial institutions. Fine-tuning the model on industry-specific data such as banking terminology, customer inquiries, and compliance requirements enhances its ability to understand and respond effectively. Similarly, models can be fine-tuned for sentiment analysis, detecting nuanced customer sentiments in product reviews or social media posts. From legal text analysis to content moderation that respects regional cultural nuances, fine-tuning makes AI truly adaptable and relevant without the need for costly, large-scale retraining.
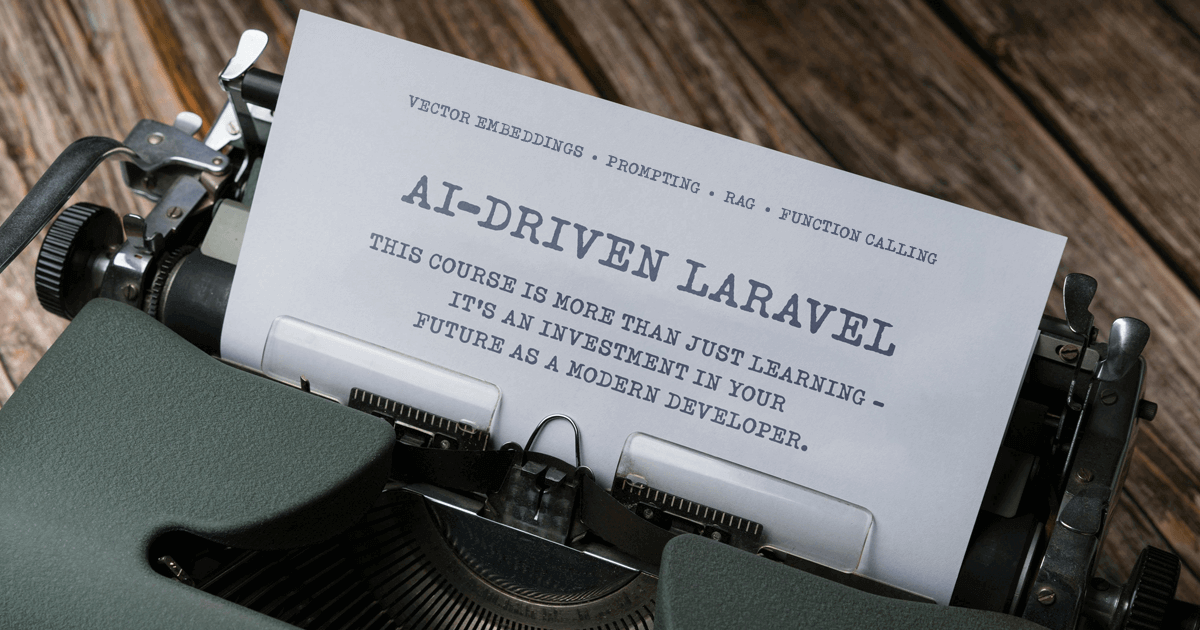
Explore More
Want to dive deeper into this and other ways AI can elevate your web apps? Our AI-Driven Laravel course and newsletter covers this and so much more!
👉 Check Out the Course: aidrivenlaravel.com
If you’ve missed previous newsletters, we got you: aidrivenlaravel.com/newsletters
Thanks for being part of our community! We’re here to help you build smarter, AI-powered apps, and we can’t wait to share more with you next week.